The CISO Framework for LLM-Powered, Agentic Security Operations
As cyber threats accelerate in speed, scale, and sophistication, traditional security operations—built on static rules, manual triage, and fragmented tooling—are falling behind. These legacy approaches lack the context-awareness and adaptability needed to respond to today’s dynamic threat landscape. The solution isn’t just more tools—it’s smarter ones. Large Language Model (LLM)-powered security agents represent a new paradigm: autonomous, context-rich systems that augment human analysts and take decisive action. They’re no longer optional; they’re your next strategic hire.
At BlueLabel, we partner with enterprises to build scalable, secure software products with an increasing focus on applied AI. This post shares an 8-step framework based on our learnings and hands-on work with clients developing LLM-powered copilots and agentic workflows for security operations.
We also recognize the natural skepticism many security leaders feel toward the idea of unleashing non-deterministic systems—like large language models—into mission-critical domains. Frankly, the idea of an unpredictable ‘AI’ making decisions in your security stack can and should cause you to reach for that bottle of Tums. At BlueLabel, we’ve long advised against fully autonomous LLM deployments that bypass human oversight. As we emphasized in our blog post on IT governance for agentic AI, safety and transparency must come first. In this blog post, we are sharing an 8-step framework, based on our leanings and hands-on work with clients, that describes how agentic LLMs can be integrated to augment human-driven security operations, not replace them.
These agents can analyze incidents, enrich context, recommend actions, and autonomously interface with existing tools. They radically improve the efficiency, responsiveness, and resilience of your security team.
But this transformation can’t happen overnight. CISOs need to build a forward-looking roadmap now: developing internal data pipelines, codifying institutional knowledge, and deploying retrieval-augmented generation (RAG) frameworks alongside custom tools, exposed via Model Context Protocol (MCP), for use by an LLM-based, security operations focused, agent. This groundwork creates a flywheel of continuous improvement where LLM-based agents become more effective over time.
The longer an organization waits, the harder it will be to catch up. The most effective way to start? A structured, actionable roadmap. Our 8-Step AI Agentic Workflow for Security Operations lays out a practical evolution—from basic ingestion to an adaptive, action-ready system.
The 8-Step AI Agentic Workflow for Security Operations
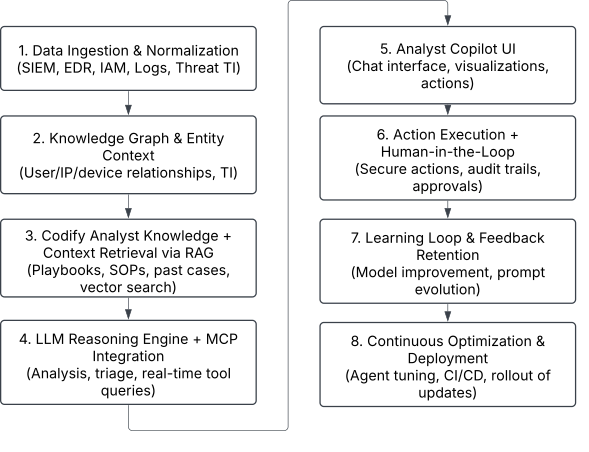
Step 1: Data Ingestion & Normalization
The foundation for deploying LLM-powered agents into security operations is structured data. The agentic workflow must ingest and normalize:
- Security Information and Event Management (SIEM) logs.
- Endpoint Detection and Response (EDR)/Extended Detection and Response (XDR) alerts.
- Identity signals.
- Threat intelligence and cloud security telemetry.
Tools: Apache NiFi, Fluentd, Logstash, Kafka, OCSF.
Step 2: Map Entities and Build a Real-Time Knowledge Graph
To reason about threats in context, the agent needs a structured understanding of your environment. This step involves constructing a dynamic knowledge graph that maps relationships between users, endpoints, identities, and actions. By connecting the dots across your infrastructure, the LLM can assess not just what happened, but who was involved and what the potential impact may be.
It helps the LLM agent understand not just what happened, but who’s involved and how it connects across systems.
Tools: Neo4j, Amazon Neptune, VirusTotal, GreyNoise, Shodan, entity resolution APIs
Step 3: Codify Analyst Knowledge + RAG Context Retrieval
Retrieval-Augmented Generation (RAG) bridges the gap between siloed knowledge and real-time decision-making—giving your LLM agents a “memory” of best practices.
Systematize what lives in your analysts’ heads:
- Incident playbooks
- Past case data
- Standard-operating procedures (SOP), enrichment logic
Codify this knowledge in structured formats (Markdown, JSON, annotated logs) and index it in a vector database. When a new incident arises, the agent retrieves relevant cases and applies institutional wisdom.
Tools: LlamaIndex, Weaviate, Pinecone, ChromaDB, Milvus.
Step 4: Enable LLM Reasoning and Integrate with Security Tools via MCP
Once the agent has context, it must begin reasoning about threats. In this step, you configure the LLM to interpret data using frameworks like MITRE ATT&CK, assess risk levels, and identify containment strategies. You also enable the agent to flag gaps in available data or situations that require human validation.
Critically, you integrate the agent with your security tools through the Model Context Protocol (MCP). MCP acts like OAuth for LLMs, providing secure, auditable, and scoped access to real-world systems like XDR, Identity Access Management (IAM), and SOAR platforms. This integration allows the agent to reason in context and prepare for responsible, supervised action.
Tools: LangGraph, Semantic Kernel, MCP adapters
Step 5: Build a Chat Interface for Analyst Collaboration
Create a secure, intuitive interface where security operations center (SOC) analysts can interact with the LLM-powered agent. This interface should serve as the command center—giving analysts full visibility into the agent’s reasoning and decision-making, while allowing for human input and oversight. There are a plethora of frameworks you can use to deploy a chatbot interface without having to build your own. Our personal favorite at BlueLabel right now is Flowise, an open-source, low-code framework for quickly building and deploying agentic chatbots.
In this step, you design the experience that builds trust: enabling analysts to chat with the copilot in natural language, review the rationale behind its suggestions, and approve or reject proposed actions before they are taken.
Tools: Flowise, CoPilot Studio, Slack/Teams integrations.
Step 6: Execute Actions with Human-in-the-Loop Oversight
Enable your agent to take high-confidence actions while maintaining human control. In this step, you configure workflows where the LLM can initiate predefined responses—such as quarantining endpoints or disabling accounts—only after a human review or via clearly governed approvals.
Actions are carried out using the Model Context Protocol (MCP), ensuring all executions are secure, scoped, and auditable. This approach allows the agent to act quickly when needed, while preserving oversight and organizational trust. Based on high-confidence reasoning, the AI agents can:
- Quarantine endpoints.
- Block IPs or domains.
- Disable compromised user accounts.
- Open tickets or notify compliance.
For a deeper discussion on how to implement the right safeguards for enabling agentic write operations in your IT environment, please refer to BlueLabels’ 6 Principles for Safely Deploying Agentic AI in Enterprise IT.
Tools: Cortex XSOAR, Tines, Swimlane, LangChain AgentToolkits, Slack/Teams-based approval bots.
Step 7: Implement Feedback Loops to Drive Continuous Learning
Turn every incident into an opportunity for your agent to improve. In this step, you build mechanisms to capture feedback from analysts—such as overrides, false positives, and resolution notes—and feed it back into the system:
- RAG databases (to inform future retrievals).
- Prompt libraries (to refine how the AI thinks and speaks).
- Orchestration graphs (to streamline next-gen workflows).
This information updates your retrieval database, refines prompt strategies, and informs orchestration logic. Over time, this feedback loop helps your LLM agent become more accurate, efficient, and aligned with your organization’s specific threat landscape and response culture.
Tools: LangSmith, Traceloop, Weights & Biases, Git-backed prompt/version control.
Step 8: Optimize and Deploy Improvements Continuously
Treat your LLM-powered agent like a product that evolves with your organization. In this step, you implement the infrastructure to continuously refine prompts, expand tool integrations, and safely deploy updates.
Establish CI/CD pipelines, governance processes, and monitoring systems to ensure the agent improves without introducing risk. As your environment and threat landscape change, your agent should adapt accordingly—without requiring a full rebuild.
Conclusion: Start Smart, Stay in Control
At the heart of this 8-step framework are two foundational technologies: Retrieval-Augmented Generation (RAG) and the Model Context Protocol (MCP). Together, they are what make LLM-based security agents both intelligent and operationally viable.
RAG provides agents with real-time access to institutional memory—allowing them to retrieve relevant past incidents, playbooks, and enrichment logic on demand. MCP ensures these insights become actionable, enabling the agent to interface securely with tools like XDRs, IAM systems, and SOAR platforms in a governed, auditable way. These two components don’t just enhance the workflow—they make it possible. Without RAG and MCP, the leap from static detection to adaptive, context-aware response is impossible.
This isn’t about launching a fully autonomous agent on day one. It’s about building incrementally—layering intelligence and capability over time, while maintaining transparency, trust, and control. Start by documenting how your analysts think. Add retrieval over historical cases. Then introduce a copilot interface, automate routine actions, and slowly expand into supervised response.
Organizations that begin this journey today—thoughtfully, with the right guardrails—won’t just modernize their SOC. They’ll build a long-term security advantage that compounds with every step forward.
Want to take the next step and explore how you can integrate LLM-driven agents in your security operations? BlueLabel helps enterprise security teams go from idea to production with real-world, resilient AI systems. Let’s talk.
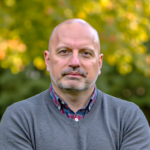